A New Approach for License Plate Detection And Localization
Security is an essential word in modern-day society. It covers many things and influences our decisions, choices, and options. One way this nebulous word affects our lives is through the surveillance of motor vehicles. If your car is stolen, police can use surveillance footage and use your license plate to identify your vehicle; they can track and locate it, possibly retrieving it before any damage is done.
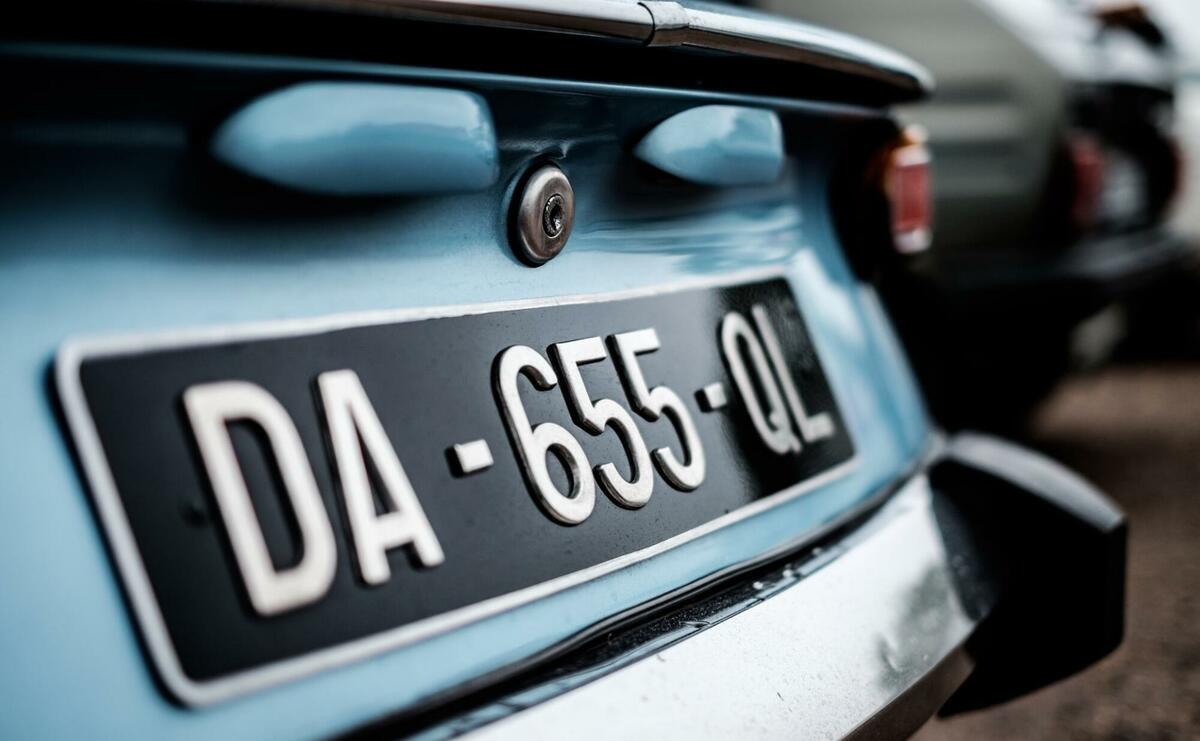
License plate detection and localization is not new and has been used for years by government offices, local and federal law enforcement, and private industry. For example, your license plate is scanned and read whenever you drive through a toll booth, and your pre-paid toll account is charged. However, this technology is expanding into dozens of other industries, and once the problems are solved, it will most likely evolve even further.
What Is License Plate Detection And Localization (LPDL)
License plate detection and localization is a technology and process that uses cameras to detect license plate numbers, extract them, and identify the vehicle. Some of the goals of LPDL are:
- Control traffic
- Witness traffic violations
- Parking lot management
- Secure area entrance management
- Automatic toll collection (EZ Pass/etc.)
- Traffic law enforcement
- Border surveillance
- Stolen vehicle search and location
- Anti-terrorism
Businesses use license plate detection and localization to track their fleet of vehicles, monitor their critical business operations, manage inbound and outbound logistics, and locate a specific vehicle to save money and improve efficiency.
However, the biggest problem these companies face is how to find a license plate from an image of a vehicle.
Challenges With Existing Techniques
Finding a license plate number can be challenging. The biggest obstacle right now is two-fold. First, the camera has to locate the license plate from the image of the car. Next, the software must extract the text from the license plate (character recognition).
Additionally, the localization piece is even more complex due to the variety of styles, shapes, sizes, colors, and textures of each state’s license plates. Detection of a license plate is the first critical step, and these issues complicate the matter making it more expensive and less reliable. In addition, license plates are not always affixed to the same location of every vehicle, making it even more difficult.
Many software applications can easily locate the plate from the image but have issues with brightness, long execution time, and accuracy. LPDL companies are working on devising better ways to improve the performance by enhancing filters and sensitivity to detect locale.
Some additional challenges with LPDL are:
- Lighting environments
- Weather conditions
- License plate placement
- License plate condition
- Vehicle movement
- Other captions (bumper stickers) in the photograph
- Image contrast
However, because LPDL is such an essential new technology, scientists are working on solutions to improve the process dramatically.
5 New Approaches for LPDL
Technologists are playing around with various techniques to improve the process of LPDL. Unfortunately, current solutions have a high error rate and are not very impressive. However, a few new methods show great promise of drastically improving the process of LPDL.
Some of the newest approaches are:
Image Acquisition
Solution engineers are toying with low-resolution versus high-res cameras to determine which makes it easier to detect a license plate from an image of the vehicle. They are testing both options in various lighting environments. The technicians are also comparing black and white photographs with full color to see which works better.
Connected Component Analysis
One proposed solution to solve the issue with localization is by running the photograph through software that uses pinpoint filtering to extract the characters of the plate. The process uses a highly sophisticated set of parameters such as the width and size of select elements around the license plate and within it.
Detecting Signature Method
Another method of handling license plate localization is running the image through software that compares a characteristic sequence of light and dark areas of the license plate, identifying it and attaching a “signature” to it. A challenge using this method is detecting which parts of the plate contain characters and which do not.
Region-Based Algorithms
Another technique is scanning an image through software connected to vast databases containing information about each local area’s license plate style, color, size, etc., to identify its localization. Testing shows that some of these region-based algorithms have a success rate of around 93.43%.
GrabCut Algorithm Method
Revolutionary scientists have developed the GrabCut algorithm as a solution for LPDL, and it shows signs of great accuracy (99.8%). The GrabCut algorithm extracts information from an image automatically without any human intervention. It uses innovative geometric information to calculate the foreground and background of an image, easily extracting the license plate data. Scientists have tested this method on more than 500 vehicles with an accuracy rate of almost 100%.
Conclusion
Vehicle plate number recognition (VPN) continues to challenge researchers, but this article and the proposed options provide hope that the process will soon be streamlined and smoother so companies may adopt LPDL into their own business activities. Once the problem of accurately detecting and localizing the license plate is solved, more companies will adopt this technology for their businesses. Local law enforcement and government agencies are already using this technology for various purposes. The only issue standing in the way is accuracy.